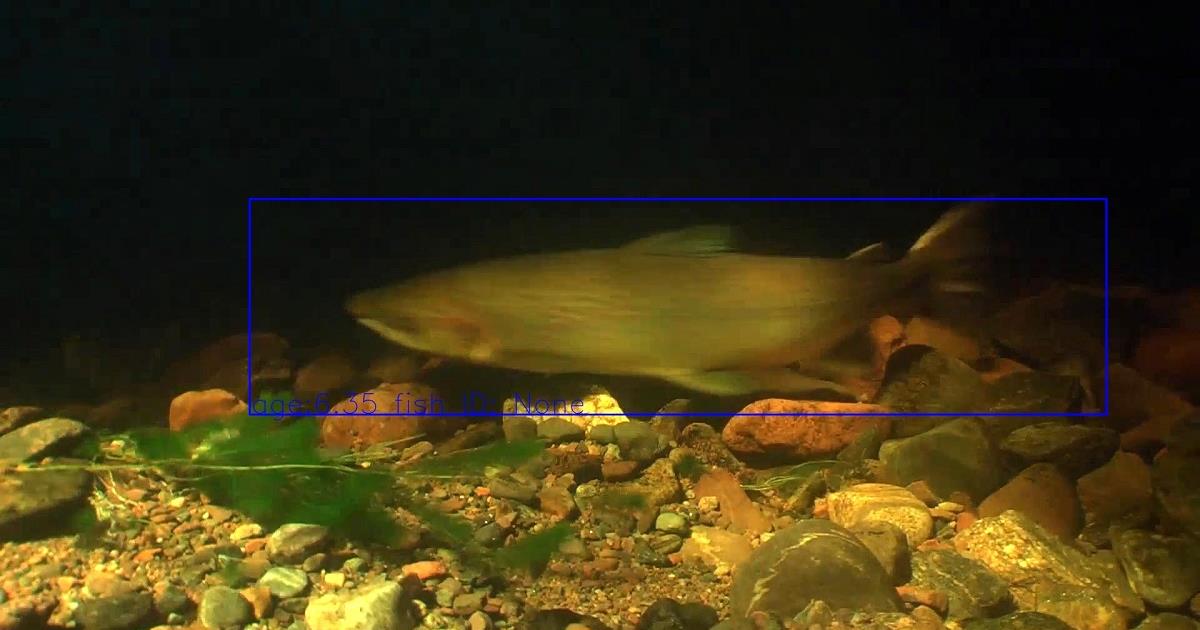
“Recording many moments containing wildlife activity presents new challenges. The most interesting clips — the ones of value to production teams — should be presented, so that producers don’t get buried by many other clips. We’ve been looking at ways to help solve this, from statistical approaches based on the metadata we generate to more abstract machine learning methods.”
Source: Matthew Judge, BBC Research & Development
AT A GLANCE:
BBC Research & Development is researching the use of artificial intelligence (AI) techniques to create intelligent video production tools. In collaboration with the BBC Natural History Unit, BBC R&D has been working closely with the Watches team (who produce the Springwatch, Autumnwatch and Winterwatch programs) to test the tools that have been developed.
Over the last three series, the system has monitored the Watches’ remote cameras for activity, logging events and making recordings of moments of potential interest.
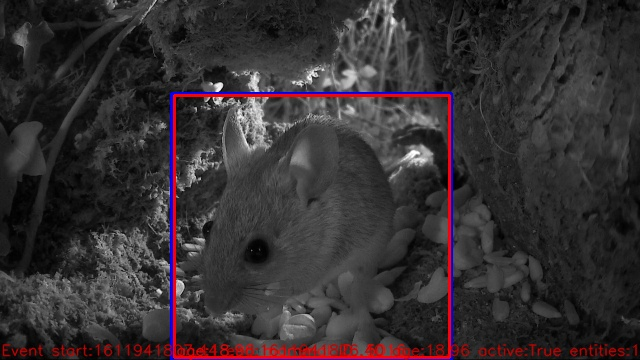
The automated camera monitoring offered is particularly useful for natural history programming since the animals appear on their own terms, and the best moments are often unexpected. It is helpful to have an automated eye watching over the live footage, to ensure those moments are captured.
A previous blog post on this subject covered the development of R&D tools for Springwatch and the shift to a cloud-based remote workflow. Since then, the team has continued to develop and polish the tools for their use in Autumnwatch 2020 and Winterwatch 2021.
EXPLORING ARTIFICIAL INTELLIGENCE:
With nearly half of all media and media tech companies incorporating Artificial Intelligence into their operations or product lines, AI and machine learning tools are rapidly transforming content creation, delivery and consumption. Find out what you need to know with these essential insights curated from the NAB Amplify archives:
- This Will Be Your 2032: Quantum Sensors, AI With Feeling, and Life Beyond Glass
- Learn How Data, AI and Automation Will Shape Your Future
- Where Are We With AI and ML in M&E?
- How Creativity and Data Are a Match Made in Hollywood/Heaven
- How to Process the Difference Between AI and Machine Learning
The team has spent time researching various areas of potential improvement. One of these areas is object tracking. While its machine learning models could detect multiple objects of interest in the scene on a per-frame basis (in this case, animals), it can be very helpful to be able to track those objects between frames. This allows the generated metadata to represent what is going on in a particular scene more clearly.
The most recent Winterwatch offered a wide variety of cameras, including the pier in Aberystwyth, the River Ness, and the New Forest. The River Ness provided the opportunity to view footage from cameras placed underwater and gave the team the chance to try its system out on fish. The team trained a new detection machine-learning model for the underwater scene, which successfully detected salmon as they swam upstream.
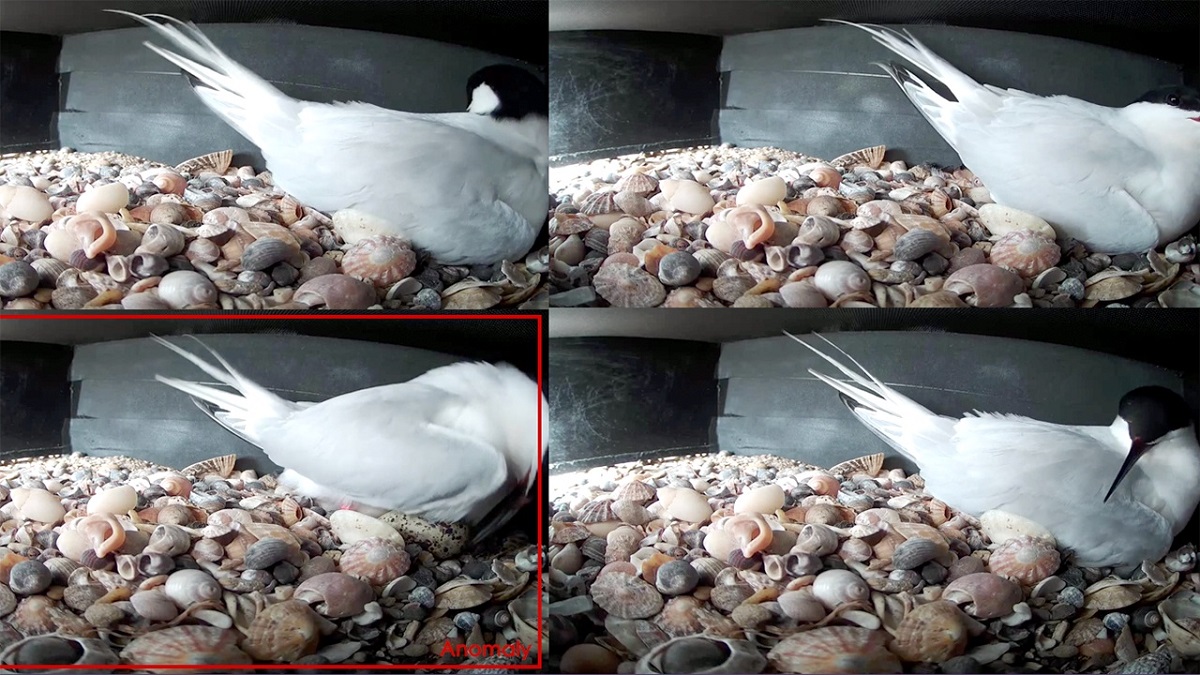
One effective approach was that of an ‘Anomaly Detector’, which was tested on Springwatch footage. Essentially, feature vectors are generated from each recording. They are evaluated to determine whether the recording fits the general trend of those previously seen from a particular camera or whether it is an ‘anomaly’, and therefore more likely to be of interest.
NOT WHODUNNIT, BUT HOW-DUNNIT — DIGGING INTO DOCUMENTARIES:
Documentary filmmakers are unleashing cutting-edge technologies such as artificial intelligence and virtual reality to bring their projects to life. Gain insights into the making of these groundbreaking projects with these articles extracted from the NAB Amplify archives:
- Crossing the Line: How the “Roadrunner” Documentary Created an Ethics Firestorm
- I’ll Be Your Mirror: Reflection and Refraction in “The Velvet Underground”
- “Navalny:” When Your Documentary Ends Up As a Spy Thriller
- Restored and Reborn: “Summer of Soul (…Or, When the Revolution Could Not Be Televised)”
- It WAS a Long and Winding Road: Producing Peter Jackson’s Epic Documentary “The Beatles: Get Back”
This approach could select clips of interest, based loosely on the animals’ actions and movement, not merely on their presence. One success story from this approach was seen when running it on footage of a pair of terns. The anomaly detector picked out clips featuring parental behavior such as turning an egg over or the brooding and non-brooding partners swapping places, which were both much more interesting than many of the other clips recorded in the tern box.
Head over to BBC Research & Development to read the full story.