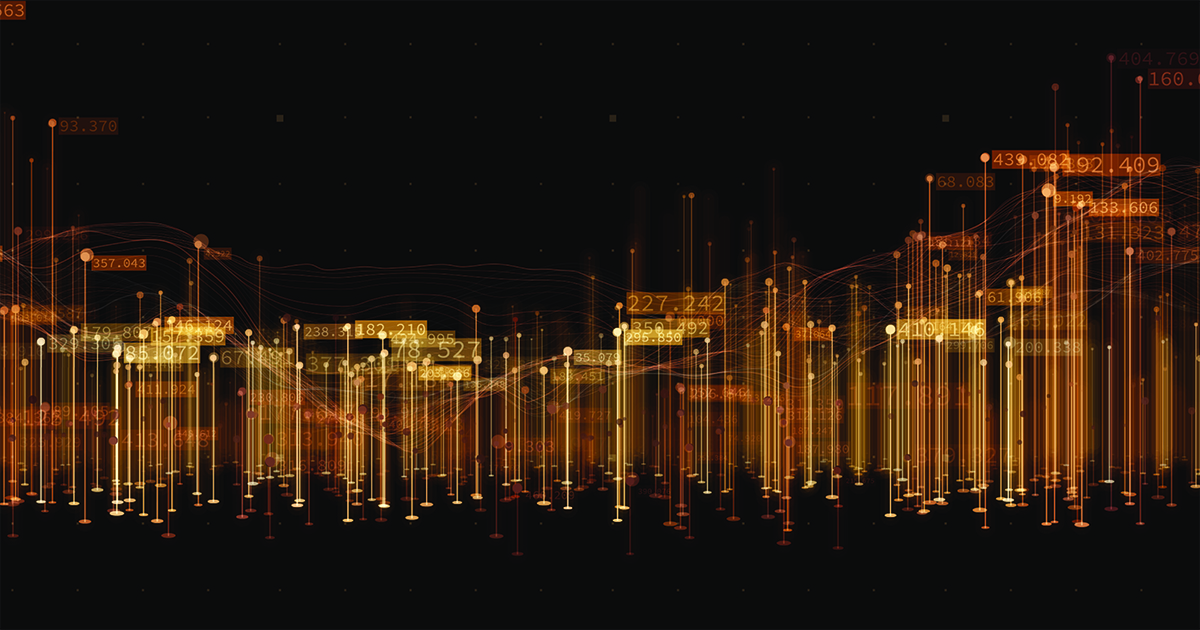
Everything is now a data point. We are all sensored. Machines are networked to the internet of things. Data collection is automated and decisions based on its aggregation are taken machine to machine in real time.
Here’s an overview of how this usage is evolving from futurist Bernard Marr — six signposts that point the direction of travel between where we are today and where data science will take us tomorrow:
AI As-A-Service
AI has been pushed into the mainstream by cloud-based as-a-service solutions, where the infrastructure is sitting in a data center and companies pay for what they use.
“The evolution of AI as-a-service means it is no longer simply helping us to automate repetitive workloads such as data entry or language translation. Increasingly it will help us make data-driven decisions such as setting strategic targets and creating smarter products and services.”
Content Creation
Marr thinks machines are increasingly giving us a run for our money when it comes to creativity, particularly in less ambitious endeavors — such as writing product descriptions or creating highlights videos for sports events.
READ MORE: What Are The Latest Trends in Data Science? (Bernard Marr & Co.)
“One huge advantage that AI has over human creatives is that the speed it can work at means it can far more efficiently produce targeted, personalized content. Product descriptions on websites can be tailored for the person that the AI predicts will be reading them, and adverts (or even movies) could have a personalized soundtrack, algorithmically created to appeal to a specific individual.”
Small Data
The events of 2020 upended many of our old customs and practices and, to an extent, wiped the slate clean on what we think we know. This means that people are now talking about “small data” — technology and practices that enable data-driven decision making — to continue when the amount of information we have is limited.
“One huge advantage that AI has over human creatives is that the speed it can work at means it can far more efficiently produce targeted, personalized content.”
— Bernard Marr
“Although it sounds like the literal opposite, small data practice is closely linked to big data concepts and will increasingly be brought into play when data becomes unexpectedly outdated due to unforeseen events or is otherwise incomplete or unavailable.”
Edge Analytics
With edge, the computational heavy lifting is carried out as close as possible to the point where the data is collected, often within the data-collecting device itself. Edge computing means decisions can be taken more quickly and reduces bandwidth taken up, sending information backwards and forwards from the cloud.
Perhaps the trend most familiar to M&E, Marr believes the edge is “undoubtedly starting to gain traction in the real world.”
Citizen Data Scientists
If data analytics are key to the survival of any company in any industry, the shortage of people who can actually understand it are a cause for concern.
“This lack of capacity to capitalize on opportunities to leverage data is undoubtedly causing a large amount of inefficiency within many organizations, purely through missed opportunities,” says Marr.
Hence, the rise of the “citizen data scientist” (a term coined by Gartner) — someone who is not necessarily academically trained as a data scientist or employed as a data analyst but has the ability to work with and implement data solutions as part of their day-to-day work.
EXPLORING ARTIFICIAL INTELLIGENCE:
With nearly half of all media and media tech companies incorporating Artificial Intelligence into their operations or product lines, AI and machine learning tools are rapidly transforming content creation, delivery and consumption. Find out what you need to know with these essential insights curated from the NAB Amplify archives:
- This Will Be Your 2032: Quantum Sensors, AI With Feeling, and Life Beyond Glass
- Learn How Data, AI and Automation Will Shape Your Future
- Where Are We With AI and ML in M&E?
- How Creativity and Data Are a Match Made in Hollywood/Heaven
- How to Process the Difference Between AI and Machine Learning
“Closely related to the AI as-a-service trend, a big driver for the explosion in popularity of this trend is the emergence of ‘no-code’ and natural-language data science platforms, allowing anyone to have a stab at creating smart applications even if they don’t know anything about software development.”
Ethical and Responsible AI
Our understanding of ethics applied to AI is evolving alongside the technology itself. It is understood that human or systemic bias can lead to automated, large-scale machine bias. “This means mitigating AI’s potential,” Marr says, since if left unchecked, AI could magnify prejudice and accelerate inequality.
READ MORE: Man In the Machine: The Ethics of AI (NAB Amplify)
AI technology is expensive, and there are only a limited number of humans with the skill to deploy it. The Partnership on Artificial Intelligence to Benefit People and Society — founded by Google, Microsoft, Apple, and others — is one organization aiming to ensure equitable AI resource sharing. Marr says most forward-thinking organizations involved with AI now often have ethics boards “dedicated to ensuring that nothing they do could be perceived as having harmful effects, and this will become increasingly common.”