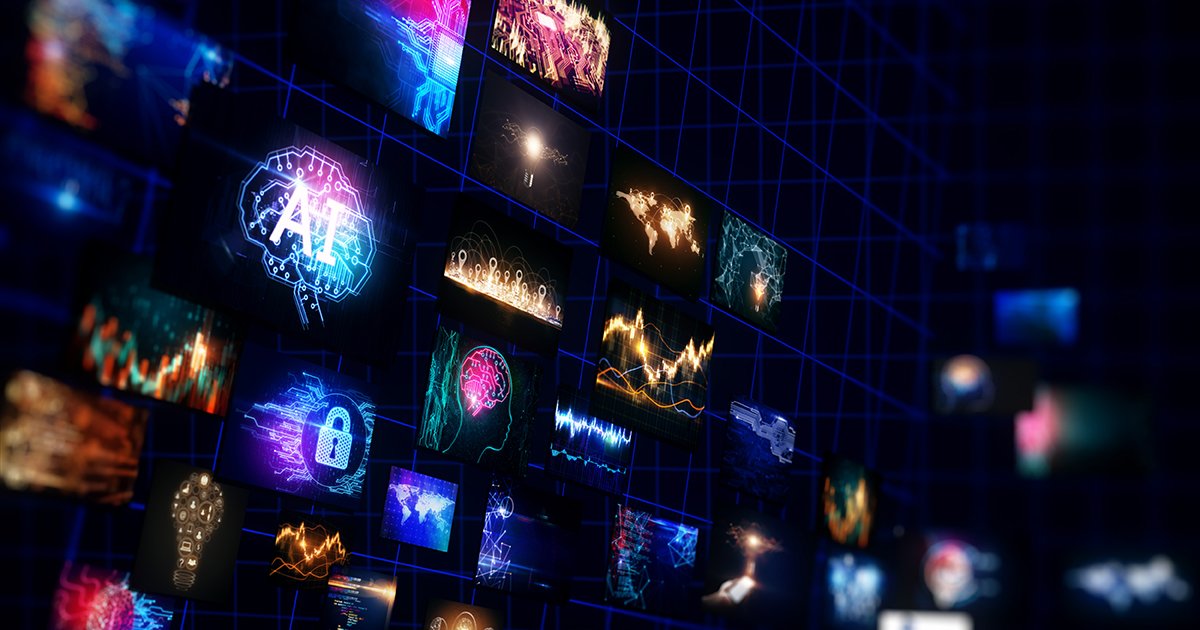
Nearly half of all media and media tech companies have adopted or are in the process of incorporating Artificial Intelligence into their operations or product lines, according to a new white paper from technology standards developer InterDigital.
“The democratization of AI toward being a fully accessible technology is underway,” the company declares.
Far from simply using AI as a buzzword or marketing tool, in 2021 media companies demonstrated solid examples of how machine learning and AI can deliver reduced cost, faster workflows, and an elevated starting position.
The white paper, commissioned by InterDigital and written by researcher Futuresource, examines the status of ML/AI across the video supply chain.
“The democratization of AI toward being a fully accessible technology is underway.”
— InterDigital
“As deep neural network training techniques develop further alongside the substantial gains in compute performance necessary to power machine learned algorithms, the applications that AI could reach and transform appear boundless,” it writes. “But there are challenges on the horizon: AI will compete with AI and, if deployed without respect, there is potential for a runaway destructive outcome that means nothing can be achieved.”
AI is now being used to help design better AI, via improved silicon or helping optimize neural networks: which will in turn lead to an acceleration of technology development.
READ MORE: AI and Machine Learning in the Video Industry: New opportunities for the entertainment sector (InterDigital)
Meanwhile, there are ethical questions surrounding AI given the challenges of AI bias on one hand and the mysterious nature of machine learned models on the other.
“There is nervousness over what happens if it works and, conversely, deep-rooted concerns over what transpires if it doesn’t work.”
Here are our edited highlights of the paper, which snapshots AI in media at the end of 2021.
Definitions
For the purposes of clarity, the paper defines Artificial Intelligence as “any human-like intelligence that is exhibited by a computer or programmable machine, effectively mimicking the capabilities of the human mind.”
Machine learning is a subset of AI that concentrates on developing algorithms and statistical models that enable machines to make decisions without specific programming.
“The machine learning process effectively refines its results and reprograms itself as it digests more data. Over thousands of iterations, this increases efficacy in the specific task that the machine-learned algorithm is destined to perform, delivering progressively greater accuracy.”
“AI-based voice recognition and transcription, face recognition, logo/brand identification, and even extraction of text from video titles and lower-thirds (for instance, subtitles on news feeds), are all being combined to create systems that can efficiently log and describe content.”
— InterDigital
And at the center of this is deep learning, or DL. This is a further subset of ML applications that can teach itself to execute a specific task autonomously using large training data sets.
“The aspect that sets deep learning apart from other methods is that these tasks are often learnt entirely unsupervised and without human intervention. By combining the three disciplines of AI, ML and DL, computers can effectively learn from examples and construct knowledge.
“The results can also surprise,” it adds.
Cost Benefit
According to the paper, most media companies view AI as enabling the automation of tasks that were not possible before. But accuracy is critical to success and expectations are high.
“AI must attain accuracy rates of 93% or above to be deemed useful; anything lower leads to distrust in its output and humans must review for quality, and the benefits are lost.
Many vendors across the media and entertainment sectors charge for AI-based tools at the point of use: they consider the length of the content being processed, or minutes of compute time required to determine the cost.
“But as the efficacy of these solutions increases over time, mirroring the uplift in AI performance, vendors may find it difficult to charge more for this improvement as the technology becomes more commonplace.”
Complexity in Training
The complexity of training required for ML typically means only the largest corporations can pay for this compute resource.
For example, the GPT-3 natural language model has more than 175 billion parameters with which to optimize its training. To put this into context, the paper estimates that a model comprising 1.5 billion coefficients costs between $80,000 and $1.6 million to train. Therefore, the 175 billion parameters of GPT-3 could cost tens of millions of dollars during training alone.
“AI is assisting producers and artists. This is not the final edit, but AI rapidly establishes an elevated starting point. AI speeds up the editing process significantly, completely automating common tasks such as finding scene transitions and cutting video into individual clips, a process that can take an experienced editor several hours.”
— InterDigital
However, even as deep learning consumes more data, it continues to become more efficient. Due to high-performance GPUs and dedicated neural network accelerators, training times have fallen drastically. Moreover, the amount of compute required to train a neural network to the same performance on image classification has decreased by a factor of two every 16 months, ever since 2012.
Bias in AI
There is concern about bias and discrimination in AI outcomes. For instance, even the most advanced facial recognition software tools sometimes incorrectly detect people of different skin tones.
“Minimizing bias in AI is crucial for the technology to reach full potential,” the paper states.
This means ensuring the original training data is diverse and includes representative examples but even this can be problematic unless the real-world data is similarly segmented.
“Even unsupervised learning is semi-supervised: after all, engineers and scientists select the training data that refines the models; and wherever humans are involved, bias can be present.”
Applications of AI in the Video Supply Chain
Across the video ecosystem, there are several applications where AI can be applied. In video encoding for instance AI’s process how to dissect and compress each image optimally by testing every possible combination and choosing the most efficient. In some video this can mean up to 42 million combinations need testing for a single video frame.
Standards organizations including MPEG JVET have begun defining new standards with AI at the center of the coding scheme, while also examining related AI techniques that can enhance existing compression schemes. Work here is likely to create an AI-based codec before the end of the decade; this has been tentatively named Deep Neural Network Video Coding (DNNVC).
The creation and management of metadata — logging — is rapidly moving from a manual procedure, often outsourced to hundreds of staff watching hours of content, to an algorithmic process.
“AI-based voice recognition and transcription, face recognition, logo/brand identification, and even extraction of text from video titles and lower-thirds (for instance, subtitles on news feeds), are all being combined to create systems that can efficiently log and describe content.”
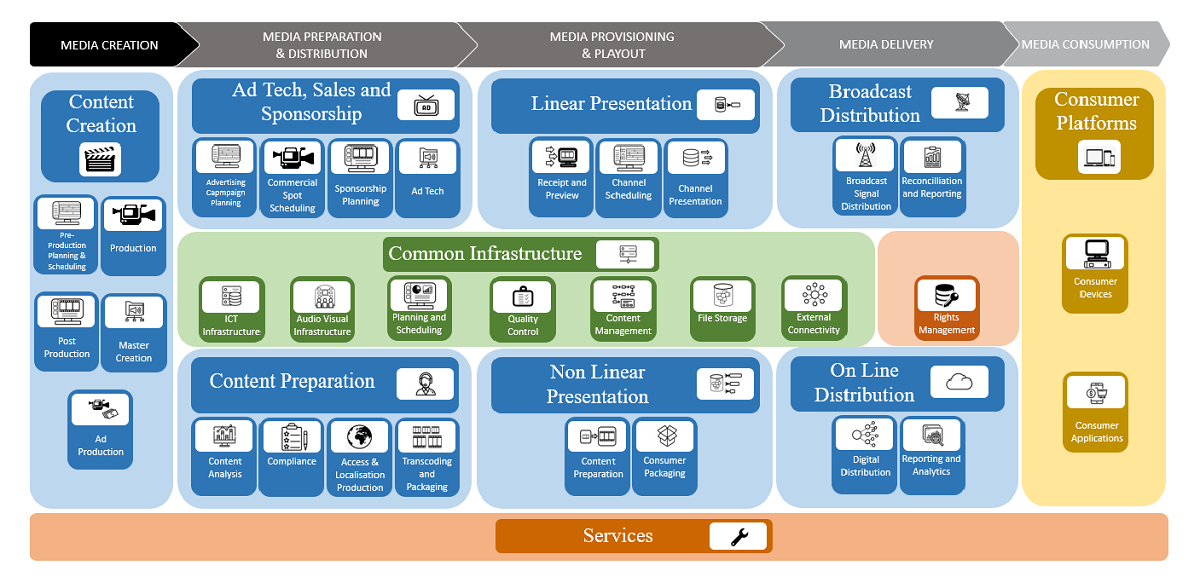
AI-driven descriptive logging also creates a more diverse archive of content with richer media assets and is routinely used to automate subtitles and generate captions in multiple languages.
“AI-based methods are now moving towards phonetic recognition systems, allowing human sounds to be recognized alongside language.”
Similar tools are being deployed for scaling content moderation. AI for image and object analysis, in addition to language transcript, allowing journalists and editors to easily detect sensitive or adult content.
ML techniques are being employed to search video for specific content, both audio and visual. Once indexed, portions of the video can be stitched together to automatically produce highlights and show reels which are immediately made available to program editors.
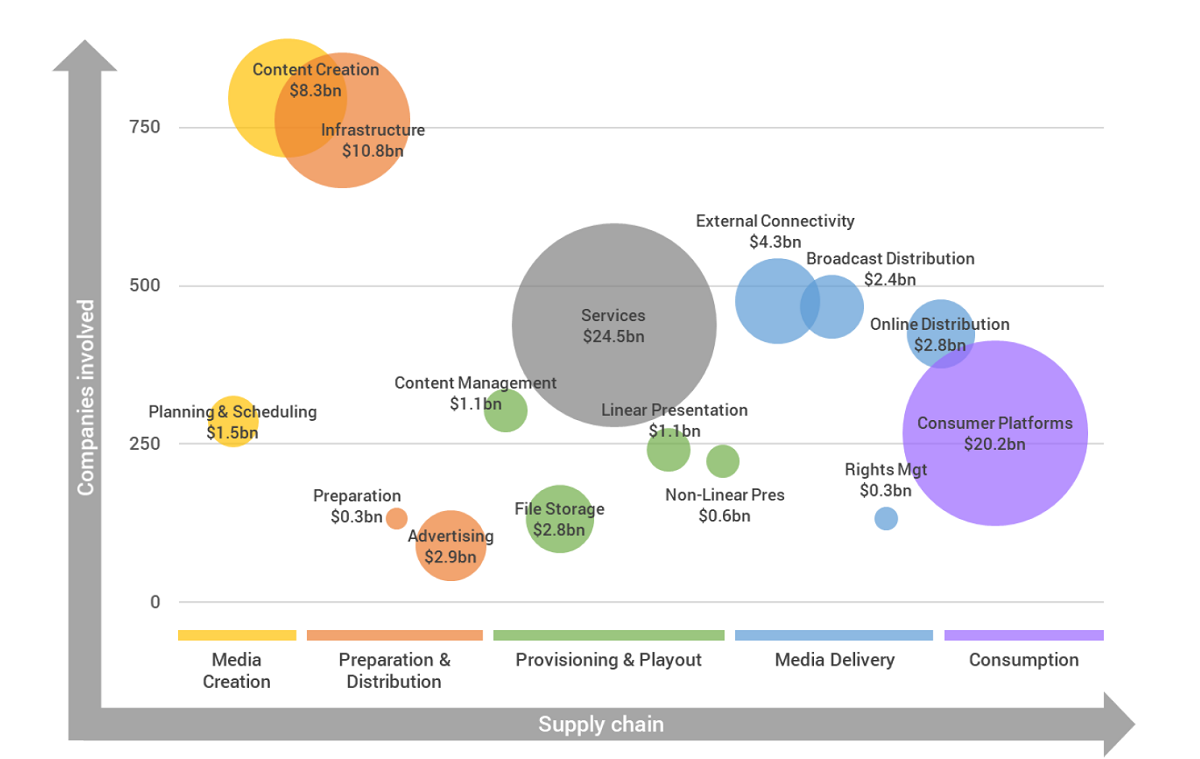
Companies like WSC Sports employ AI to analyze what happens during sports games, identifying each and every point, and isolating the most compelling moments. They then create automated short-form highlights and video clips for social media with minimal human involvement during editing.
Auto-reframing and frame rate correction tools are also available in editing software such as Adobe Premiere Pro and Blackmagic Design’s Davinci Resolve.
“AI is assisting producers and artists. This is not the final edit, but AI rapidly establishes an elevated starting point. AI speeds up the editing process significantly, completely automating common tasks such as finding scene transitions and cutting video into individual clips, a process that can take an experienced editor several hours.”
AI and ML are solving quality problems such as the identification of lip-sync issues. There are even AIs able to match scenes across films and apply appropriate color grades to visually similar clips during editing, dependent on parameters set for the content and mood.
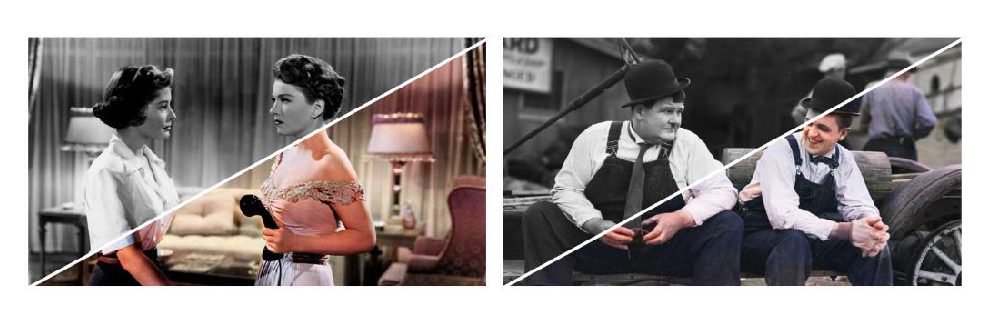
AI VFX
The digitization of humans using AI is gaining traction: notably, face-swapping technology leverages machine learning’s ability to convert one image into another. By doing so, producers can transfer performances from one person to another; for instance, stuntmen are easily replaced using AI-based video processing to transform them into creatures or Hollywood actors.
AI further offers the opportunity for fully automated digital human likenesses. LG’s keynote speaker at CES 2021, Reah Keem, was a virtual human.
READ MORE: Getting Real with Virtual Influencer Reah Keem (LG Newsroom)
Fable Studios creates “virtual beings” using digital character generation technologies, bringing human representations to life through AI visuals, voice dialogue and animation.
READ MORE: Giving Characters Life With GPT3 (Fable Studios)
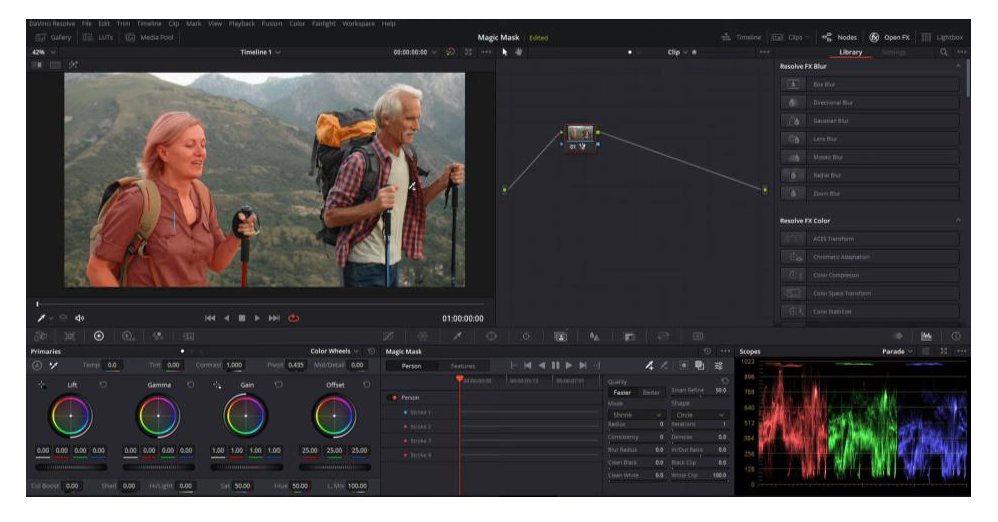
Deepfakes: A Threat and An Opportunity
ML is used to determine which media gets promoted in news feeds on Facebook; which videos are recommended on YouTube; what content appears at the top of timelines in Twitter, and more. And the effect of this is potent: AI algorithms now heavily influence what gets shared and followed by humans.
Media outlets worldwide are trading on the honesty and accuracy of their journalism; integrity is a vital element, since it underpins how their content is perceived by the public, and trust directly affects business. But today, AI is capable of fabricating utterly convincing, yet completely untruthful, text, images and video: this is the world of “deepfakes.”
“The consumption of intentionally created false or manipulative content is seen as an imminent danger. As video is now a primary means of communication, there is a threat that relates to the use of AI for misinformation and exploitation, which could have an impact on the foundations of society.”
AI Will Compete with AI
A solution is to build detection systems that quickly identify deepfakes whenever they appear, but the challenge here is that AI will compete with AI, and as performance increases so will the requirement for better detection.
Alternative strategies call for some form of digital certification scheme. Digital fingerprints or watermarks are not always reliable; however, a blockchain online ledger system could hold a tamper-proof record of videos, pictures and audio, so that their origins can be verified, and any manipulations discovered.
“Along with other synthetic media and fake news, the more sinister impact of deepfakes is to create a zero-trust society where people cannot distinguish truth from deception. Once trust is eroded, it is easier to raise doubts about specific events. So, it’s entirely feasible that media outlets might actively seek AI-based tools that allow detection and discovery of disingenuous media.”
Futuresource’s research maintains that the human eyeball is essential in helping detect deepfakes and further ensuring quality control in all forms of media; unsurprisingly, humans are very adept at quickly identifying anomalies and irregularities.
Copyright
Governments are beginning to contemplate the implications of AI and copyrights. Generally, they are examining the use of copyrighted works and data by AI systems themselves, whether copyright exists in works generated by AI and who it belongs to, and how to extend copyright protection for AI software and algorithms.
This is gaining attention because machine learning systems learn from any data made available to them; this might include works protected by copyright such as books, music and photographs, as well as digital assets published online. This means that the copyright owner’s permission is required by law to use any part of the work unless a copyright exemption applies.

It is entirely feasible for AI to avoid copyright infringement by using licensed or out-of-copyright material. For instance, an AI could be trained using the works of Shakespeare, which are no longer protected by copyright. But unless a work is licensed, out of copyright, or used under a specific exception, an AI will infringe by making copies of it, or by creating derivations from the original.
AI-based training might infringe copyright, however AI-based search can also alleviate copyright and content licensing issues.
“Along with other synthetic media and fake news, the more sinister impact of deepfakes is to create a zero-trust society where people cannot distinguish truth from deception. Once trust is eroded, it is easier to raise doubts about specific events. So, it’s entirely feasible that media outlets might actively seek AI-based tools that allow detection and discovery of disingenuous media.”
— InterDigital
For instance, AI-based methods are enabling musicians who are not receiving compensation from the market to receive royalties for their content. Indeed, as music streaming services dominate the industry, producers apparently receive only around $1 for every 10,000 views or playbacks.
AV Mapping, a Taiwanese-based start-up, offers AI technology to discover copyright-free and licensable audio assets for television and film making. In this incarnation, the AI helps content makers avoid the time-consuming process of browsing through studio music catalogues, testing music in the edit suite, and examining the licensing terms. Instead, the solution analyses the video being produced, including the edits and cuts throughout, compares the results to a large music database, then recommends audio assets appropriate to each section of the video.
A New Landscape of AI Regulation
“There are certainly real policy questions around AI. Legislators are afraid about what bad actors could do with AI and are equally concerned about mistakes made by AI-based decision-making. There is nervousness over what happens when AI works as intended, likewise anxieties over what happens when it fails.
EXPLORING ARTIFICIAL INTELLIGENCE:
With nearly half of all media and media tech companies incorporating Artificial Intelligence into their operations or product lines, AI and machine learning tools are rapidly transforming content creation, delivery and consumption. Find out what you need to know with these essential insights curated from the NAB Amplify archives:
- This Will Be Your 2032: Quantum Sensors, AI With Feeling, and Life Beyond Glass
- Learn How Data, AI and Automation Will Shape Your Future
- What’s the Endgame for AI?
- How Creativity and Data Are a Match Made in Hollywood/Heaven
- How to Process the Difference Between AI and Machine Learning
The challenge is that there are no general laws that can regulate AI, and ultimately it may become necessary to draft specific laws for different applications. AI techniques will be an integral element in future systems, so writing a law attempting to cover AI in general might prove to be at the wrong level of abstraction.”
Future Prospects
The neural technologies underpinning AI will no doubt expand as machine learning becomes ever more accessible. Indeed, large corporations are now building massively parallel computer chips and components precisely architected to accelerate cloud-based AI and ML.
Meanwhile, consumer products are now able to execute AI inferencing tasks entirely locally on device thanks to systems on chip (SoCs), which combine powerful GPUs with Neural Network Accelerators dedicated to offloading computation of neural tasks from the central processor. This is most evident on smartphones, which use AI for computational photography, object and face recognition, AR, and voice recognition among others.
“These chips are becoming so complex to create, so much so that AI is actually being used in some instances to help design them!”
The Intersect with Quantum Computing
Quantum computers being developed by Google, Intel, IBM, Microsoft and Honeywell represent the next evolution of today’s fastest Supercomputers. These machines are expected to solve presently intractable problems in science and business, with applications spanning chemical modelling to drug discovery.
IBM debuted a 65- qubit “Hummingbird” processor last year and, by 2023, the company plans to release a 1,121-qubit quantum processor called “Condor,” which it believes marks the inflection point where businesses will finally be able to reach the era of “quantum advantage.”
“As deep neural network training techniques develop further alongside the substantial gains in compute performance necessary to power machine learned algorithms, the applications that AI could reach and transform appear boundless. But there are challenges on the horizon: AI will compete with AI and, if deployed without respect, there is potential for a runaway destructive outcome that means nothing can be achieved.”
— InterDigital
By 2030, IBM plans to install a quantum computer that contains one million qubits. This represents the world’s most ambitious, concrete plan for a quantum computer — at least among those that are publicly known. Google is also believed to constructing a quantum computer of similar capability, to be operational before the end of the decade.
“Potentially, perhaps by the end of the decade, we look towards the intersect between Neural and Quantum computing. This combination promises a monumental shift in compute performance, with complex problem-solving on completely new levels; indeed, the capabilities presented by ‘Bits + Qubits + Neurons’ will transform experiences and deliver even more intelligent machines.”